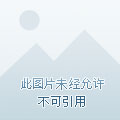
Reporting by XinZhiyuan
EDIT: LRS
【New Zhiyuan Introduction】Face recognition technology has recently had a new way to crack! A Stanford student used a GAN model to generate several pictures of himself, easily breaking through two dating apps, and the most outrageous thing was that "women dressed as men" could not be recognized.
Someone can really imitate your face and bypass the face recognition system!
Recently, researchers at Stanford University published a paper on arxiv, although it is a coursework of Stanford CS236G, but the paper proposes a very interesting idea, using adversarial generation network GAN to generate a facial image to imitate the target face, to see if the face recognition system can be correctly verified.
Because the key feature information of the face is retained, the results of the paper show that the resulting face image can still be verified by the face, which is simply a big defense for those systems that rely only on flat image recognition.
Thesis link: https://arxiv.org/pdf/2203.15068.pdf
The researchers tested the black box in the face verification system of two dating apps, easily overcoming the face verification process, and even after converting the female face to a male, it can still be verified by the face.
According to the authors, the work is the first attempt to bypass facial verification using the resulting image, where the original face image has specific recognition features but another, drastically altered identity can also be verified.
Whose face is this?
After the online verification system is breached, all kinds of fraud can have very negative consequences, so people are particularly interested in detecting and combating imposters.
Unlike ordinary authentication, face verification involves verifying the declared identity based on the face image, the face and identity are one-to-one matches, but once there is another completely different face that can open the same lock as you, how do you prove that "I am me"?
At present, many mobile apps claim that face verification technology is safe and can protect the user's identity, such as Bumble and Tinder and other dating software identification process is the user using the built-in camera to take a photo, and then compare it with the photo in the user's profile.
There is a big problem with this type of use of photos for face detection: a fake photo that bypasses the face recognition system can be verified.
If you want a face with the same characteristics as the original face, but look different, the adversarial generative network model is perfect.
But since the birth of GAN, how to control the generation of images consistent with expectations, or to guide the generation process of GAN models through a feature vector, has been a major challenge in the GAN subliminal space.
While techniques and tools such as gradient-weighted class activation mapping (Grad-CAM) can help establish potential directions between classes and enable transformations, if you look further at the resulting images, you can see that such models have very limited control over the fineness of the transformations.
Experimental methods
The authors used two datasets as a basis for their experiments:
One is a dataset of human users, consisting of 310 images of the authors' faces, spanning four years, with different light, age and perspective, extracted from the cropped face through Caffe;
The other is the 108501 images in the FairFace dataset after category balancing, which were also extracted and cropped.
In order to verify the experimental effect locally, the researchers built a face verification model locally, mainly using a ConvNet Inception pre-training model on the basis of FaceNet and DeepFace, and the training of image vectors used triplet loss, where A is an anchor image, P is a positive example, N is a counterexample, and α is an interval.
This validation model uses facial images from a training subset of FairFace, and in order to pass face validation, the Evolutionus norm distance is calculated between the input image and the target user in the database, and any image below the 0.7 threshold is equivalent to the same identity, otherwise the validation is considered a failure.
Image generation model, the author directly used the StyleGAN model, fine-tuned on the personal data set, randomly generated images can be directly through the local face verification system, and the picture looks really different from the picture in the training dataset.
The weights of the first four layers are frozen when fine-tuned to avoid overfitting of the data to produce an image of a face that is too similar to the training dataset.
Although images different from the original face can be obtained with a basic StyleGAN model, the results of the baseline model are similar in quality to the images of the training dataset (less diverse) and have lower resolution (low fidelity).
The authors' second attempt was to use the StarGAN v2 model, which allowed to train seed images on target faces.
To prevent overfitting, the StarGAN v2 model was pre-trained for about 10 hours using the FairFace validation set. To generate images, the authors also tried to use the training data as a seed image and the processed image of a human user dataset as the source image.
The authors also tried to use the processed images of the personal user dataset as seed and source images, but the results did not improve much.
In the verification process, the author first randomly selected 1000 face images to find out which faces passed the local test verification, and then tested whether the images generated using GAN could be rematched.
The following figure shows the author photo on the left, the photo that failed to verify in the middle, and the photo that succeeded in verification on the right.
The purpose of the experiment was to create as large a gap as possible between perceived visual identities while retaining the decisive features of the target identity, using the Mahalanobis distance as an evaluation indicator, which is also an indicator commonly used in image processing for pattern and template searches.
For the baseline generation model, the low-resolution results obtained showed less diversity despite passing local face validation, while StarGAN v2 was able to create more diverse validation-pass images.
The resulting images were tested on the face verification systems of dating software Bumble and Tinder, taking the image as the author as a baseline, and successfully passed the verification.
The "male" version of the author's face also passed Bumble's verification process, although the light had to be adjusted in the resulting image to be accepted, while Tinder was smarter and didn't get fooled.
These are groundbreaking experiments with identity projection in the context of GAN subliminal manipulation, which remains an extraordinary challenge in the study of image synthesis and deep forgery. This work also opens up the concept of continuous embedding highly specific features in different identities, as well as the creation of "alternative" identities to "read" other people's identities.
The devil is one foot tall, and the road is one foot high
Nowadays, in people's daily lives, "brushing face" is very common, such as shop passenger flow statistics, uninhabited container brush face payment, unit access control, family door locks, bus/road security monitoring, company face recognition attendance, express parcel pickup, bank card opening, online payment, hotel accommodation and other fields are widely used "face recognition" technology, and showing an expanding trend.
Face recognition technology can currently be divided into two categories: based on 2D face images and based on 3D face images. 2D face recognition shoots flat imaging through 2D cameras, so even if the algorithm and software are advanced, under limited information, the security level is not high enough, and it is easy to be cracked through photos.
As early as 2019, some primary school students held up photos to "crack" Fengchao's face recognition system.
The 3D face recognition system with a high level of security through the 3D camera stereoscopic imaging, generally there will be 4 probes, two of which are large cameras, the other two are infrared probes for filling light, one is a visible light probe, and the two cameras cooperate with each other to form a 3D image, thereby restoring the complete three-dimensional world. Current 3D face recognition technology can accurately distinguish photos, videos, masks and twins.
At present, there is also a crucial technology in the face recognition identity authentication system that is widely used - live detection, that is, the system camera correctly identifies whether the face is in person at the same time, and checks whether someone uses photos and other means to impersonate a legitimate user. This is also why when the bank "brushes the face", it is often necessary for users to complete the "left look right look", "blink" and other actions.
The devil is one foot high, the road is one foot high, and only by constantly fighting, the technology will continue to develop upwards.
Resources:
https://www.unite.ai/creative-facial-verification-with-generative-adversarial-networks/